While traditional sales strategies were once built on intuition and experience, the modern sales landscape has evolved.
Companies that thrive today leverage data-driven insights to make informed decisions. T
his is where predictive sales analytics comes into play.
Predictive sales analytics has been somewhat of a buzzword for a while, but huge innovations have been made in recent years, changing the game. Predictive sales analytics allows sales teams to go beyond merely reacting to market trends; it empowers them to get ahead, anticipate changes, and drive revenue growth.
In this blog, we’ll explore the intricacies of predictive sales analytics, recent innovations in the field, how it works, and the tangible benefits it offers to sales managers and organizations.
Predictive Sales Analytics: The Basics
Sales analytics refers to using data analysis and statistical techniques to gain insights into sales performance and forecast future sales outcomes. At its core, sales analytics involves collecting and analyzing data related to sales activities, customer behaviors, market trends, and other relevant factors that can impact sales outcomes.
Streamline commissions for your RevOps, Finance, and Sales teams
Design, track, and manage variable incentives with QuotaPath. Give your RevOps, finance, and sales teams transparency into sales compensation.
Talk to SalesRecent Innovations in Predictive Sales Analytics
As we said, sales analytics has come a long way over the past decade. Driven by advancements in technology, data science, and artificial intelligence, it has evolved significantly and now offers a lot more benefits for sales teams.
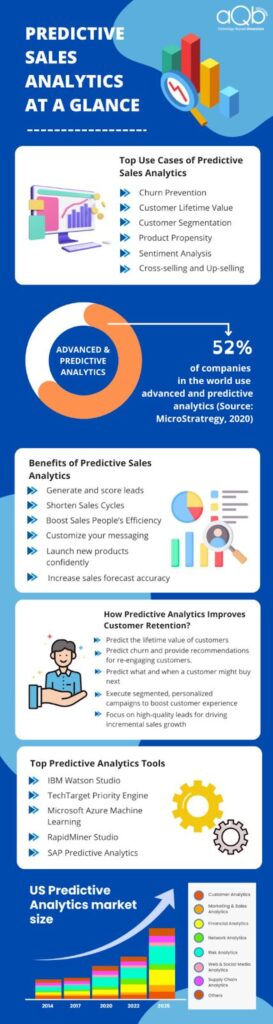
Two of these innovations stand out for their transformative impact on the field: AI-Powered Sales Forecasting and Predictive Lead Scoring.
AI-Powered Sales Forecasting
AI-powered sales forecasting is revolutionizing the way sales teams predict future performance and form a long range plan. Traditional sales forecasting relied heavily on historical data and often involved manual adjustments based on intuition. However, these methods had limitations, particularly in rapidly changing markets or when unexpected variables came into play.
With AI-powered sales forecasting, these limitations are a thing of the past. AI algorithms can process enormous amounts of data from multiple sources. These algorithms analyze complex relationships within the data to produce highly accurate sales forecasts.
One of the key advantages of AI-powered forecasting is its ability to learn and adapt continuously. As more data becomes available, the AI model updates its predictions, making the forecasts increasingly accurate.
Predictive Lead Scoring
Predictive lead scoring is another game-changing innovation in sales analytics.
In traditional lead scoring, sales teams often relied on predefined criteria to evaluate and prioritize leads. These criteria might include demographic information, past interactions with the company, and the lead’s position in the sales funnel. While useful, this approach could be subjective and might not always accurately reflect a lead’s true potential to convert.
Predictive lead scoring, on the other hand, leverages machine learning algorithms to analyze a much broader set of data points. These include behavioral signals, past purchasing patterns, engagement levels, and social media activity.
The AI model evaluates these factors to predict which leads are most likely to convert into customers, assigning a score reflecting the conversion likelihood. With this insight, sales teams can prioritize these high-potential leads, focusing their efforts where they are most likely to yield results.
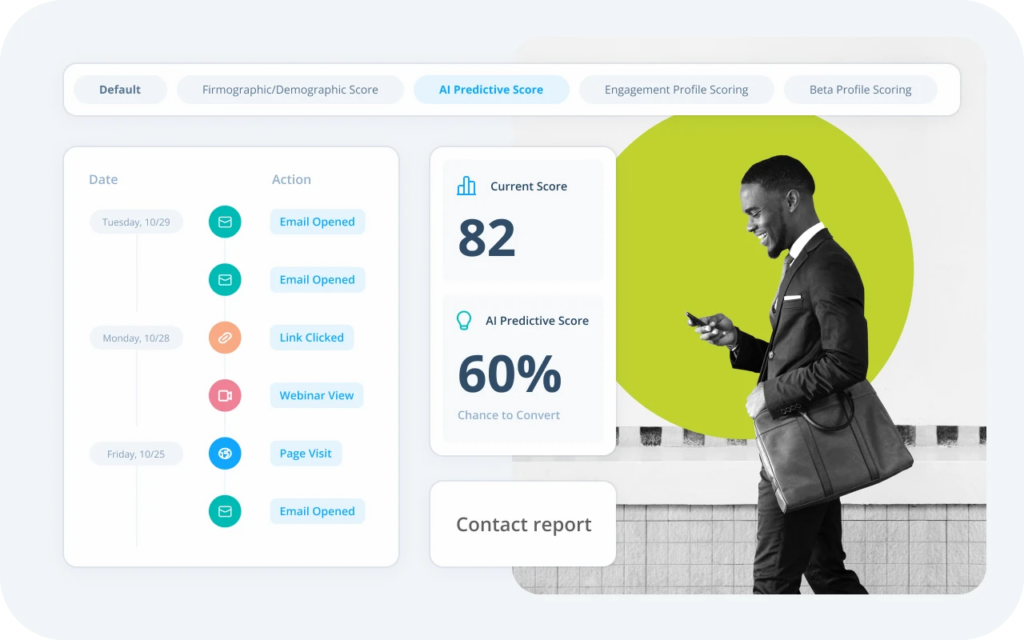
How Does Sales Analytics Work?
Predictive sales analytics is an essential tool for modern sales teams, and like any tool, to get the most out of predictive sales analytics, it’s helpful to have a rough idea of how it works.
Predictive sales analytics operates through a systematic process that transforms raw data into actionable insights, enabling sales teams to anticipate future outcomes and refine their strategies accordingly. The workflow involves several critical stages, each playing a vital role in ensuring the accuracy and relevance of the predictions made.
- Data Collection
The foundation of predictive sales analytics is comprehensive data collection. This involves gathering data from a wide array of sources, like CRM systems, historical sales records, marketing campaigns, social media interactions, and customer feedback.
Additionally, external data such as market trends, economic indicators, and competitive intelligence is incorporated to provide context. The more diverse and detailed the data, the more accurate and insightful the predictive models will be.
- Data Integration
Once data is collected, the next step is integration. Data from different sources is often stored in various formats and locations, making it necessary to bring all this information into a unified system. This can be especially challenging when working with a legacy financial system.
Data integration ensures that all relevant information is available in one place, creating an easily accessible, holistic view of the sales landscape. This unified dataset is crucial for making connections across different data points and for accurate predictive modeling.
- Data Cleaning and Preparation
Before analysis can begin, the raw data must be cleaned and prepared. This involves identifying and correcting errors, removing duplicates, and resolving inconsistencies. For instance, missing data points might need to be imputed, and outliers that could skew results may be addressed.
Data preparation may also involve transforming the data into a suitable format for analysis, such as normalizing data or categorizing continuous variables. Clean, well-prepared data is critical for the accuracy and reliability of predictive models.
- Data Analysis
With clean and integrated data, the analysis phase begins. This is the core of predictive sales analytics, where advanced statistical models, machine learning algorithms, and AI tools are applied to the data.
These techniques identify patterns, trends, and correlations that might not be immediately apparent. For example, machine learning algorithms can uncover hidden relationships between customer behaviors and purchasing decisions or identify factors that consistently precede successful sales.
The complexity of the models can range from simple linear regressions to sophisticated neural networks, depending on the nature of the data and the specific predictive goals.
- Insight Generation
The analysis phase produces a wealth of information, but the value lies in translating these findings into actionable insights. Predictive sales analytics generates insights to forecast future sales trends, predict which leads are most likely to convert, identify potential risks such as customer churn, and recommend strategic changes.
These insights enable sales teams to make data-driven decisions that are proactive rather than reactive. They can be hugely beneficial when developing a go to market strategy for startups, SMEs, and product launches for larger corporations.
- Visualization and Reporting
To make the insights generated from predictive analytics actionable, they need to be communicated effectively to decision-makers. This is where visualization and reporting come in. Predictive sales analytics tools often include dashboards and visualizations that present the data in an easily digestible format.
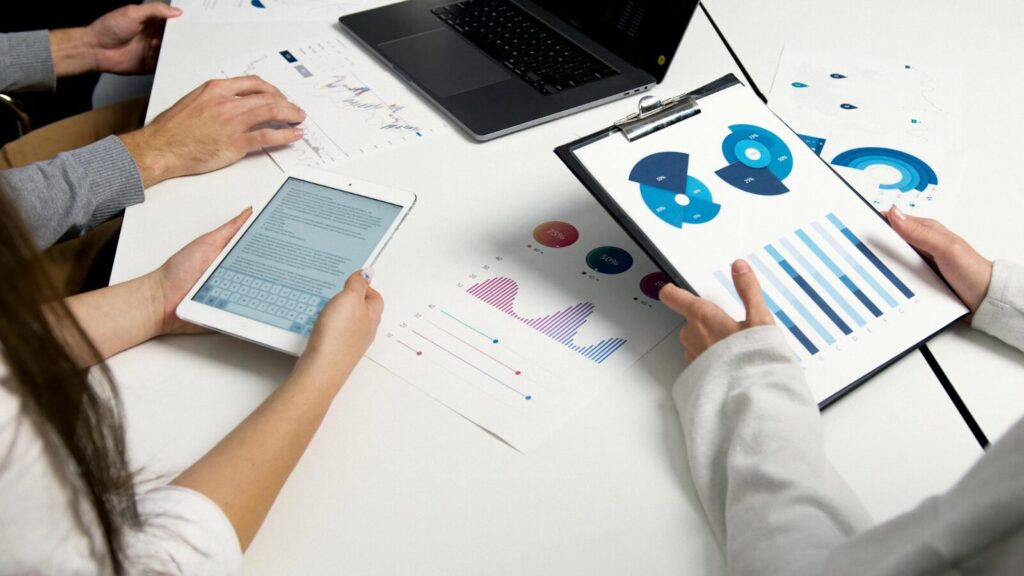
These visualizations might include graphs, charts, heat maps, and other visual aids that highlight key trends and predictions. Reports can be customized to focus on specific metrics or segments, providing sales managers with the information they need to make informed decisions quickly.
- Implementation
The final step is implementation, where the insights derived from predictive analytics are put into practice. This might involve adjusting sales strategies, such as focusing on high-potential leads identified through predictive lead scoring, optimizing pricing strategies based on demand forecasts, or reassigning resources to areas predicted to yield the highest returns.
The implementation phase is crucial, closing the loop between data analysis and tangible business outcomes. Regular monitoring and iteration based on ongoing data analysis help ensure that strategies remain effective and aligned with the ever-changing market dynamics.
What Are the Benefits of Sales Analytics?
Predictive sales analytics offers transformative benefits that can significantly impact a company’s revenue optimization and overall sales strategy. By leveraging advanced data analysis techniques, sales teams can move beyond traditional reactive approaches to become more proactive, strategic, and data-driven in their decision-making.
Here’s an in-depth look at the key advantages of predictive sales analytics.
- Improved Sales Forecasting
Predictive sales analytics significantly enhances the accuracy of sales forecasting by analyzing sales data, market trends, and external factors.
Traditional forecasting methods often rely on simple historical trends or educated guesses, which can lead to inaccurate predictions. In contrast, predictive analytics uses complex algorithms and machine learning models that can process vast amounts of data to identify patterns and correlations that might not be immediately obvious.
By incorporating these diverse data sources, predictive analytics can produce more reliable and nuanced forecasts. This allows sales managers to set realistic sales targets, anticipate fluctuations in demand, and make informed decisions about resource allocation based on business profitability analysis.
Streamline commissions for your RevOps, Finance, and Sales teams
Design, track, and manage variable incentives with QuotaPath. Give your RevOps, finance, and sales teams transparency into sales compensation.
Talk to Sales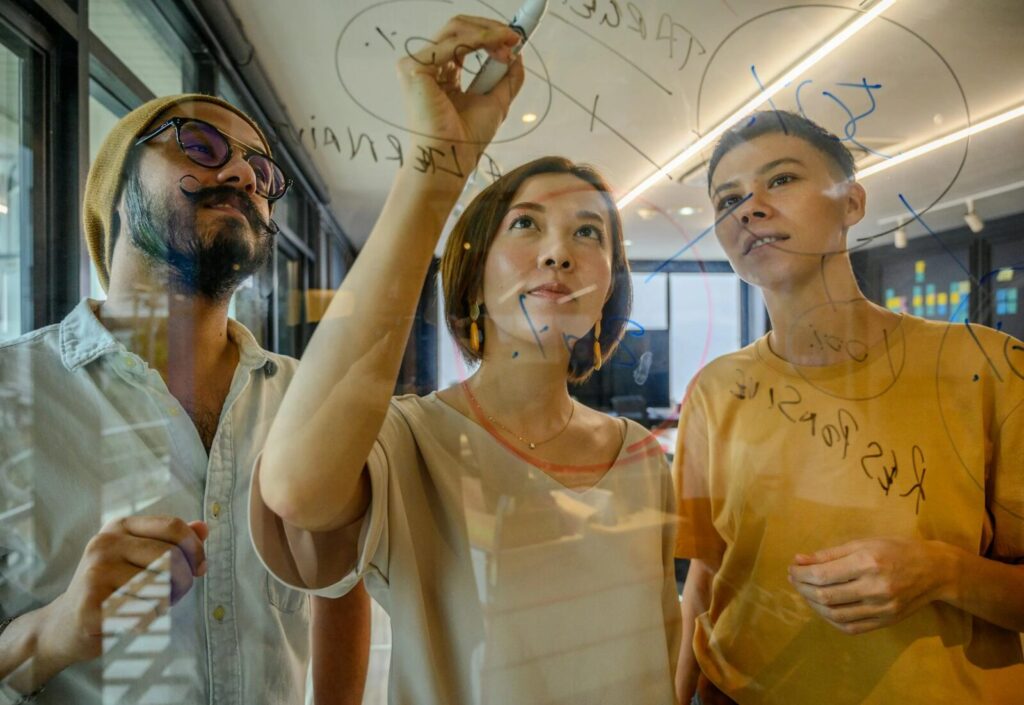
- Enhanced Customer Targeting
One of the most powerful applications of predictive sales analytics is its ability to enhance customer targeting. By analyzing past customer behavior, demographics, purchasing patterns, and engagement levels, predictive analytics can identify which customers are most likely to make a purchase or respond positively to specific sales strategies.
This allows sales teams to focus their efforts on high-potential leads and customer segments, thereby increasing the efficiency and effectiveness of their sales campaigns. High-value customers might receive personalized offers or premium services, while those at risk of churn might be targeted with retention campaigns.
- Personalized Sales Strategies
A one-size-fits-all approach is no longer sufficient in today’s highly competitive and customer-centric market. Predictive sales analytics enables the development of personalized sales strategies (such as these ABM marketing examples) by providing deep insights into individual customer preferences, behaviors, and needs.
By analyzing data such as past purchases, browsing history, social media interactions, and feedback, predictive models can suggest the best ways to engage with each customer. So, if predictive analytics identifies that a particular customer frequently purchases certain products at specific times of the year, sales teams can proactively reach out with personalized offers or recommendations before the next anticipated purchase.
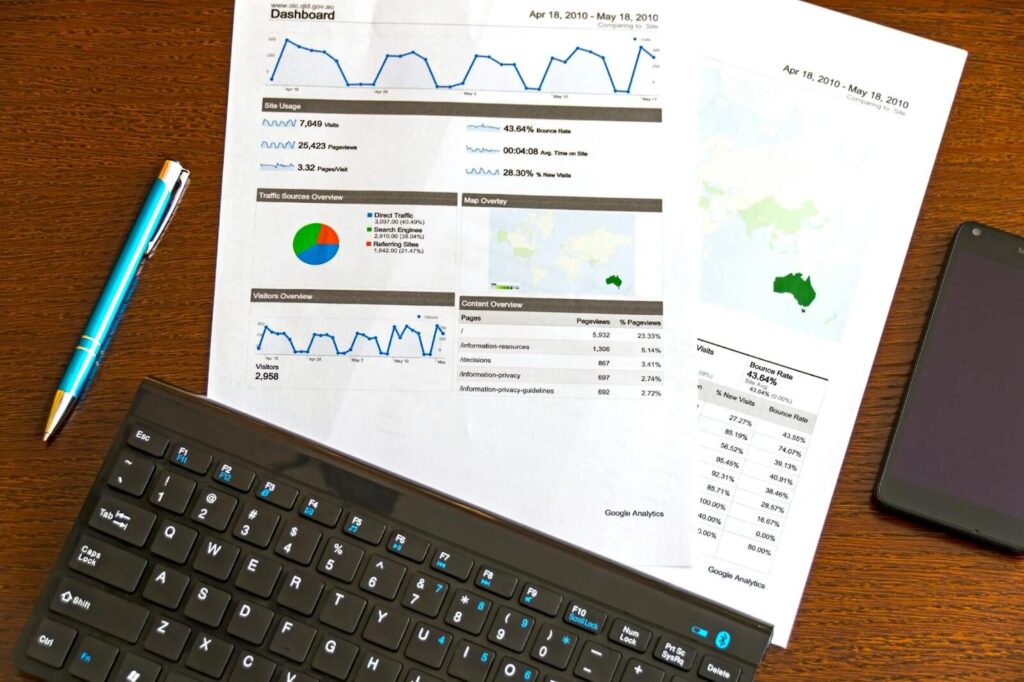
- Optimized Pricing Strategies
Pricing is a critical factor directly impacting a company’s sales volume and profitability. Predictive sales analytics is crucial in optimizing pricing strategies by analyzing how different pricing models affect customer behavior and sales outcomes.
Traditional pricing strategies often rely on basic cost-plus approaches or competitor benchmarking, which may not fully capture the complexities of customer demand. Predictive analytics, however, can model the relationship between price changes and sales volume, considering factors like customer segments, seasonal demand, and competitor actions.
By identifying the optimal pricing strategy for different scenarios, predictive analytics helps companies maximize revenue and profitability.
- Increased Sales Efficiency
Efficiency is key to maximizing the productivity of a sales team and scaling finance operations, and predictive sales analytics offers significant improvements in this area.
By identifying the most effective sales activities and strategies, predictive analytics allows sales teams to focus their efforts where they will have the most significant impact. This might involve prioritizing high-potential leads, refining the sales pitch for different customer segments, or concentrating on the sales channels that deliver the best results.
Additionally, by automating the identification of high-value opportunities and optimizing the allocation of resources, predictive analytics reduces the time and effort spent on less promising leads.
- Proactive Sales Management
Another standout benefit of predictive sales analytics is that it empowers proactive sales management. Rather than reacting to problems as they arise, sales managers can use predictive insights to anticipate and address potential challenges before they become a problem. This proactive approach is particularly valuable in identifying early signs of declining customer engagement, potential market shifts, or emerging competitive threats.
For instance, with Onestream’s FP&A, sales managers can identify a downward trend in engagement from a key customer segment, signaling a potential drop in future sales.
With this insight, sales managers can implement targeted campaigns to re-engage these customers before the problem escalates. Similarly, if predictive analytics forecasts a slowdown in a particular market, sales managers can adjust their strategies or redirect resources to more promising areas.
Try QuotaPath for free
Try the most collaborative solution to manage, track and payout variable compensation. Calculate commissions and pay your team accurately, and on time.
Start TrialKey Takeaways
Predictive sales analytics is transforming the way sales teams operate, offering a wealth of opportunities to optimize revenue and drive growth. By leveraging data-driven insights, sales managers can improve forecasting, enhance customer targeting, personalize sales strategies, and make better-informed decisions.