This is a guest blog from the data and AI company, Databricks.
Sales forecasting is supposed to make everyone’s lives easier. Sales and marketing have their targets. Customer services and accounting understand workloads and expected revenue. Despite this, a report found that 68% of companies miss their sales forecast by more than 10%.
Why such a huge discrepancy? Businesses aren’t using the right data or nearly enough of it.
AI lead scoring is a strategy that improves forecast accuracy and empowers your organization with more efficient sales and marketing activities.
In this article, we’ll explain how AI lead scoring can benefit your business.
Earnings & Attainment Forecasting
Learn how QuotaPath provides reps with current and forecasted earnings and attainment views.
Talk to SalesWhat is AI Lead Scoring?
AI lead scoring uses machine learning (ML) and predictive modeling to rank sales prospects. Data such as demographics and customer behavior train AI models. The AI then identifies the trends and patterns of your target audience. AI lead scoring drives marketing and sales optimization.
Data processed in AI lead scoring includes:
- Customer personal information
- Firmographics such as company size
- Engagement with emails and marketing campaigns
- Social media interactions
- Psychographic data such as interests and cultural beliefs
- Loyalty data, including purchase history and customer loyalty metrics
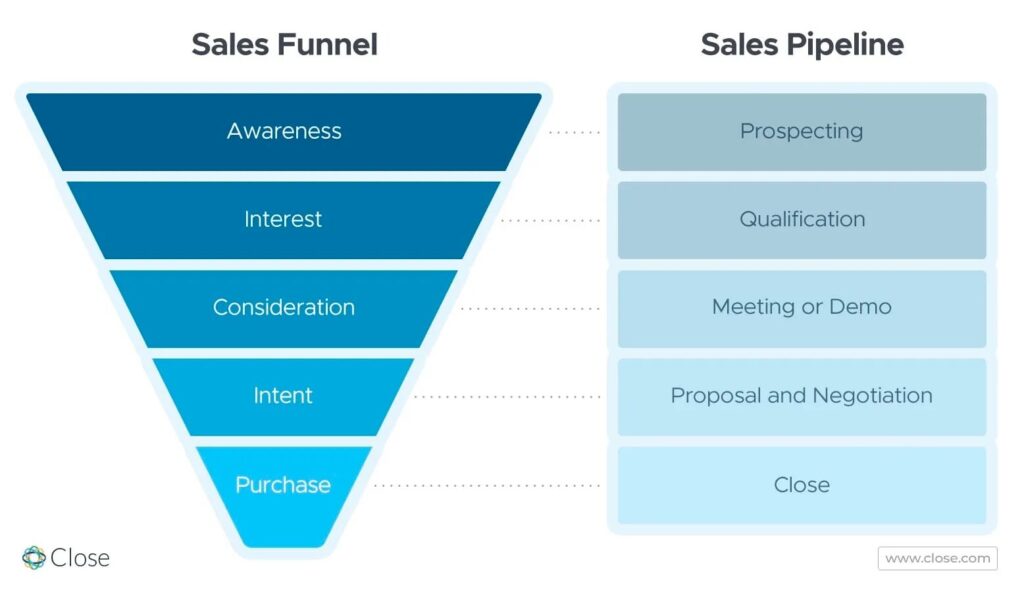
Image sourced from close.com
Using this information, the AI algorithms quickly identify the potential of each lead by assigning them a score. Lead scoring turns your sales pipeline into a cascading sales funnel of momentum.
Traditional vs AI Lead Scoring
Traditional lead scoring has been around for about as long as the sales funnel has been a concept. It requires a hands-on, manual process from both sales and marketing professionals.
Much of the scoring information comes from lead magnets. What is a lead magnet in marketing? It’s that free ebook or email newsletter you get prospects to sign up for.
Team members must seek out categorical information and rank it against baselines before assigning a score. The baselines typically come from ideal customer profiles (ICPs). Much of the scoring is down to the subjectivity of the person doing the ranking, and liable to human error.
Because AI uses historical data for accurate results, AI-based solutions allow organizations to automate and improve efficiencies in the sales pipeline.
AI lead scoring reduces the need for salespeople to be involved in the ranking process. It helps to eliminate human error, emotion, and bias from lead scoring.
Let’s look at a breakdown comparing AI and traditional lead scoring:
Traditional Lead Scoring | AI Lead Scoring | |
Scalability | Increasing workloads require more staff and more time. | Can process large data volumes and scale with need. |
Accuracy | Accuracy depends on user subjectivity and the available data. | Eliminates biases, pulls from more data sources, and identifies hidden patterns. |
Adaptability | Lead scoring must be manually adjusted and updated. | Learn and adapt over time. Data is processed in real time to reflect changing market trends and consumer preferences. |
Performance | A manual process that takes up your team’s time. Increasing the number of leads causes bottlenecks. | Once AI is properly trained, lead scoring is fully automated. Increasing the number of leads does not affect performance. |
The benefits of AI Lead Scoring
It takes considerable effort to set up an AI lead-scoring strategy. Is the ROI worth it?
Let’s take a look at some AI lead-scoring benefits:
- More accurate, less biased lead scoring: AI calculates without emotion or prior influence. The algorithm connects the dots to find quality leads that your team might miss.
- Reduced human error: automation reduces errors associated with manual entry such as duplicates.
- Increased flexibility: train models with new datasets to adjust for changing market trends. Lead scoring also scales with need without increasing the workloads on your team.
- Saved time: automation reduces the need for manual lead scoring, increasing sales productivity. Your sales and marketing teams can spend more time engaging potential customers and growing your pipeline.
- Increased profits: your team focuses on the best leads for higher conversion rates.
- Integrated with business tools: connect your CRM, ERP, and other platforms for analysis and more precise modeling. Use scoring data in real time for more accurate demand planning and sales forecasting.
- Streamlines processes: AI not only enhances sales-related processes but also reduces manual workloads across various departments, such as inventory management and accounts payable, improving overall operational efficiency.
- Lower costs: eliminates manual scoring, reducing your labor overheads.
How AI optimizes sales forecasting
Sales forecasting is vital to your business’s strategic planning. It helps you better allocate resources and budget expenditures based on revenue projections.
So how does predictive AI improve forecasting?
Precision of data-driven insights
AI lead scoring requires large volumes of data. While this can take some initial work, it pays off in dividends. Behavioral analytics offer new insights into your target audience and how to better engage with them.
Predictive analytics also produce opportunities such as personalized marketing. AI-powered insights help your team tailor each interaction for a better customer experience and higher win rates.
Record every touchpoint for more accurate lead scoring and forecasting.
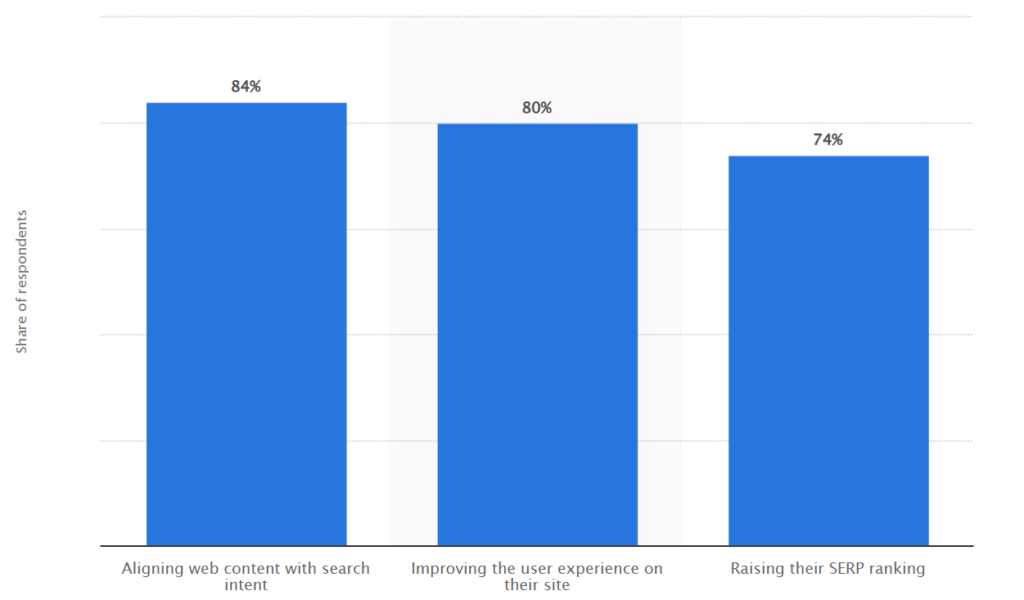
For example, a recent survey of marketers identified aligning web content with search intent as the most effective use case for AI. With automated lead scoring, you can discover which pages to audit and which to leave alone.
Let’s say you have a landing page for your certification for data engineers course. Automated lead scoring shows that most visitors are data scientists, creating a mismatch. Your marketing team takes this insight and audits the page for better SEO keywords.
You can flag the lower-performing pages for search engine optimization using data-driven insights. You can consider your new SEO strategy when generating your next sales forecast.
Better lead scoring
AI models identity patterns from data your sales team simply doesn’t have access to or the bandwidth to process. For example, website user behavioral data connects to your AI lead scoring. The algorithm identifies high-quality leads based on their browsing behavior.
Better lead scoring will improve your sales numbers. It will also give you a more accurate idea of what’s happening in your funnel. Use this data to bolster your short and long-term sales forecasts.
Connection between marketing and sales
Lead scoring provides a pulse check on your sales pipeline. With human error and bias eliminated, there’s no one to point the finger at. AI lead scoring aligns marketing and sales with the cold, hard facts.
Accurate lead scoring gives both teams a common system and promotes inter-departmental collaboration. To maximize the benefits of this alignment, it’s often necessary to hire marketers who are skilled in interpreting AI data and executing effective strategies. This can help increase lead quality and improve sales forecasting is in each department’s best interests.
Real-time predictions
AI lead scoring begins as soon as a prospect enters your pipeline. With each interaction, their score changes, becoming more precise.
All ranking data feeds into other business tools, such as sales forecasting. Over time, models adapt for greater accuracy, sending better data to those tools. In other words, the more data your AI lead scoring analyzes, the more accurate it becomes at predicting sales.
It also helps you make more money. A recent survey found that 80% of global businesses saw increased revenue after implementing real-time data.
Try QuotaPath for free
Try the most collaborative solution to manage, track and payout variable compensation. Calculate commissions and pay your team accurately, and on time.
Start TrialHow to implement predictive lead scoring with AI
Customers interact with your brand in a wide variety of ways. When they enter the pipeline, you’ve already got ample information about their likelihood to make a purchase. However, you need to learn how to crack the code and decipher customer intent.
How do you put together a lead or account scoring strategy led by predictive AI?
- Identify target audience
Sales team marketing tactics begin with knowing your target audience. Collaborate with your marketing and sales teams to create ideal customer profiles. You can base ICPs on market research and historical data but there may be a bit of guesswork at the beginning.
What type of characteristics make up your ideal customer? Create specific details for each profile based on the following criteria:
- Demographics: age, gender, education, income, occupation, household size, geographic location
- Firmographics (B2B): company size, turnover, revenue, industry, existing tech stack, location
- Lead behavior: touchpoint interactions, marketing engagement, past purchase history
- Psychographics: values, attitudes, interests, buying habits
- Pain points: what challenge does your product solve for this customer?
For example, let’s look at an ICP for a company that provides marketing and sales automation:
- Businesses with 100+ employees
- Annual turnover exceeding 10 million dollars
- Multiple outbound sales channels
- Three-month buying decision process
- Ability to call international numbers with a local presence
Remember these are ideal customer profiles. Not every lead will be a perfect fit. It’s still up to identify the best opportunities—that’s where lead scoring enters the picture!
- Gather data
You can’t start ranking new leads without any data. What sources of information will help you (or the AI) determine the value of a lead?
Build a data collection strategy that aligns with your ICPs, pulling in the right data from every relevant channel.
Some sources for lead scoring data include:
- Websites: track visitor activity while interacting with your web pages. Tracking pixels and cookies identify repeat visitors. You can also use website forms to gain contact information and other demographic details. Heatmaps and session recordings add another level of insight into user behavior.
- Business tools: customer relationship management (CRM) software and other apps are great sources.
- Social media: use a social media analytics tool to record prospect engagement rates and gather firmographic data. Incorporating a social media post generator can automate content creation, ensuring consistent engagement and data collection.
- Content marketing: email marketing tools and other solutions enable you to track clicks, open rates, and conversions for every prospect.
- Lead source: the origin of each lead is vital to lead scoring. Organic website traffic will have a different win rate than a customer referral. If historical data is scarce, consider using a Bayesian Neural Network (BNN) to make the most out of every dataset. You will use this data to train and evaluate your predictive model later on.
- Spam detection: not every interaction adds up to a positive. Some leads can be bots or disinterested users. For example, a freelance writer may have no use for your sales dialer, but they do want access to your most recent industry report.
- Segment your leads
The goal of any lead scoring system is to evaluate potential customers for fit and interest. We can divide this into four basic categories: avoid, Stimulate Interest, Follow up, and Take Orders.
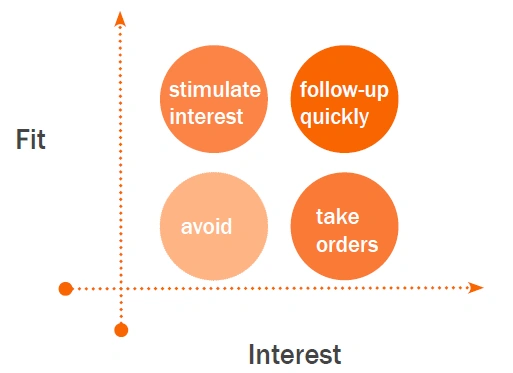
The beauty of lead scoring is that you get a more granular classification of every lead, streamlining customer segmentation. A typical system uses scores from 0 to 100 or 0 to 1. You can usually adjust this to what works best for you.
Prioritize scoring criteria in order of what you think is most valuable. Give more weight to the top factors by making them worth more points. As you move down the list, decrease the weight score for each category.
If every box is ticked on an ICP, the lead score should add up to 100.
- Set a lead score threshold
Your ideal customers are just that, ideal. These types of businesses or individuals are the unicorns your sales and marketing teams dream of. Here in the real world, you compromise on what prospects to spend your time and money on.
Looking at the data so far, it’s time to set a minimum lead score. This will be the threshold for the “Avoid” category. Any lead that falls below this score can’t be ignored and forgotten.
Start with a low threshold. This ensures you don’t miss out on opportunities, and soon, the predictive AI will hone in on an optimal score.
- Train your AI model
Okay, so you have the foundation of a lead scoring system in place. The good news is that you aren’t going to rely on your team to manually tally up every individual score. It’s time to train your predictive AI.
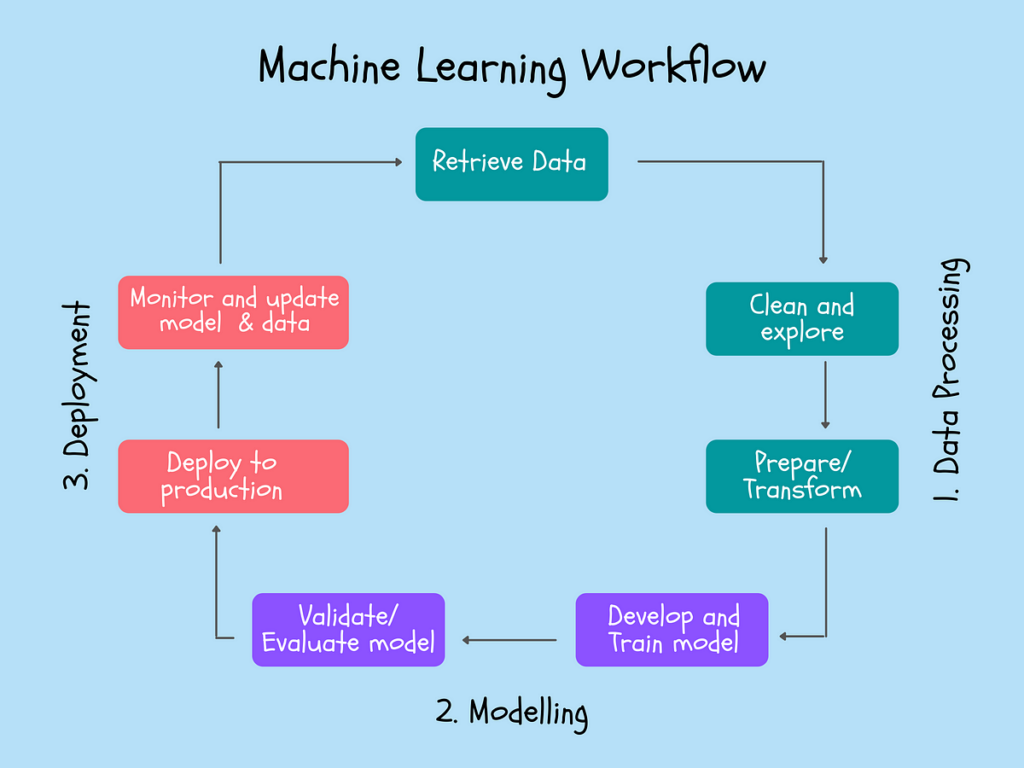
Use separate data sets to train your AI. The first set will teach the AI as it looks for patterns. You will use the second set to test the accuracy of your trained AI.
The AI training process for lead scoring is as follows:
- Gather historical customer and market data and form two sets of data.
- Clean and process the data, removing duplicates and errors.
- Train the AI with dataset one.
- Test the AI with dataset two and evaluate for accuracy.
- Deploy the model and start generating lead scores.
- Monitor results and adjust
Now your AI lead scoring model is putting out scores nearly instantaneously. Sounds great, right? However, it’s time to evaluate the results. Use your CRM and sales analytics tools to monitor the results.
Key performance indicators (KPIs) to track include:
- Conversion rate
- Win rate
- Lead-to-opportunity ratio
- Sales velocity
- Revenue
- Customer lifetime value
- Lead score accuracy
Establish a baseline for every metric before implementing an AI solution. Compare the results. If performance is decreasing or staying put, it’s time to re-evaluate your lead scoring model.
Use AI Lead Scoring to win more sales
Growth is great. When your business increases its total sales, everyone is busy. However, you don’t want to stretch your team out too thin. AI lead scoring ensures every prospect is rated fairly and without bias, saving your team valuable time.
You can focus your resources on the best opportunities while preventing potential buyers from slipping away. Setting up an AI lead scoring system for your business takes time, but it’s worth it in the long run.